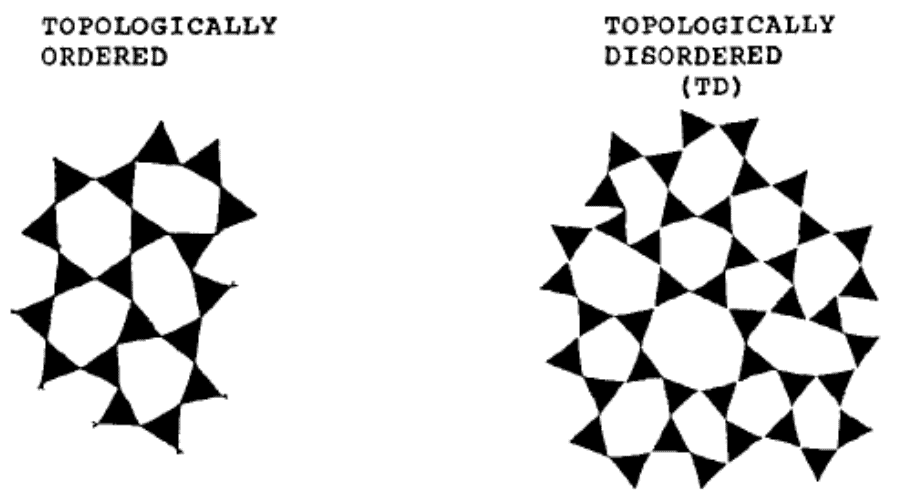
[Image above] Schematic of glass networks exhibiting (a) topological order and (b) topological disorder. Credit: Gupta, Journal of the American Ceramic Society
This month’s topic of the Glass: Then and Now series, Glass topology and artificial intelligence, is a natural follow-up to last month’s post on Molecular dynamics simulations. Both topology and MD are modeling techniques aimed at defining the structure of glasses and designing compositions for specific performance characteristics.
While molecular dynamics uses interatomic potentials to arrive at structural insights, the technique requires substantial time and computing power for each iteration. This limits its usefulness for compositional studies and property predictions currently.
In his 1932 paper, W.H. Zachariasen at University of Chicago provided a model of glass structure, which has since been expanded by a number of researchers into “topological constraint theory.” This model consists of a polyhedral of atomic arrangements (short-term order) connected randomly according to a set of topological (geometric connectivity) constraints that allow for the formation of a network with long-range disorder.
Topological constraints are based on the allowable geometries of bonds between two atoms and bond angles among three bonded atoms. The restrictions of the bonds are compared to the degrees of freedom for atomic motion. Glasses form when these two are in balance. However, when there is either too much or too little freedom of motion, the system can crystallize.
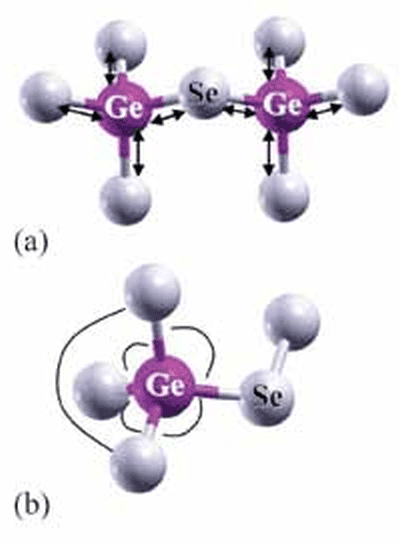
The structural possibilities for the polyhedral and associated constraints along with their temperature dependencies are often characterized by techniques such as nuclear magnetic resonance. Molecular dynamics is frequently used in the absence of such measurements.
Mathieu Bauchy, associate professor of civil and environmental engineering at the University of California, Los Angeles, is a leader in the fields of topological constraint theory and artificial intelligence. He is teaching the upcoming ACerS live online course, Machine Learning for Glass Science and Engineering, October 17–19. Click here to learn more about the course and register.
The following information is excerpted from Bauchy’s recent article, “Analytical model of the network topology and rigidity of calcium aluminosilicate glasses,” which describes the technique and its usefulness for the calcium aluminosilicate glass system. Another excellent resource for understanding topological constraint theory is “Topological constraint theory of glasses” by John C. Mauro, ACerS Bulletin, May 2011.
“The structure and properties of the calcium aluminosilicate (CAS) ternary system have been extensively studied from experiments, atomistic simulations, and machine learning. Because the stoichiometry of CAS and other oxide glasses can be continuously adjusted, there exists a largely untapped opportunity to discover new glass compositions featuring desirable properties and functionalities. However, the vastness of the accessible compositional space renders traditional material discovery approaches (e.g., Edisonian ‘trial-and-error’) inefficient.
To this end, topological constraint theory (TCT) has been a key enabler to develop predictive models that relate the composition and structure of glasses to their properties. Various TCT-based models have been proposed over the past decades to predict glass-forming ability, glass-transition temperature, liquid fragility, hardness, stiffness, dissolution rate, etc.
The success of TCT is based on the fact that many macroscopic properties of disordered materials primarily depend on the topology of the atomic structure, whereas other structural details only have a second-order effect. As such, TCT reduces complex disordered atomic networks into simpler structural trusses, wherein some nodes (the atoms) are connected to each other by some topological constraints (the chemical bonds).
In structural glasses, topological constraints comprise of the radial two-body bond-stretching and angular three-body bond-bending constraints. The number of constraints per atom then offers a simple, reduced-dimensionality metric that is often correlated with macroscopic properties.
Importantly, predictions from TCT critically rely on an accurate knowledge of the glass structure and connectivity, which is the key to enumerate the number of bond-stretching and bond-bending constraints created by each type of atoms in the glass network. This is a challenge as the local structure of glasses (and hence, the number of constraints) changes as a function of composition.
For instance, CAS glasses exhibit several structural complexities, for example, overcoordinated aluminum atoms, tricluster oxygen units, free oxygen species, varying calcium environments, etc. All these features impact the constraints enumeration and, hence, should be accounted for in robust topological models. Although such information can be accessed by molecular dynamics (MD) simulations of one composition at a time, it is not practical to systematically conduct MD simulations over large compositional domains considering their high computational cost.
In addition, discrete models (e.g., relying on a finite, discrete number of MD simulations) are not differentiable, that is, they do not allow for the computation of the derivative of the number of constraints per atom with respect to composition. This prevents the use of gradient-based “inverse design” optimization methods (e.g., to pinpoint glasses with minimum, maximum, or tailored rigidity).
Here, to address these challenges, we present a fully analytical model describing the network topology of (CaO)x(Al2O3)y(SiO2)1−x−y (CAS) glasses over the entire ternary domain. This model is informed and validated by a series of classical MD simulations but, importantly, offers a pathway to continuously predict the properties of CAS glasses as a function of their compositions without the need for any systematic MD simulation.”
– Mathieu Bauchy, University of California, Los Angeles
Articles for Glass topology and artificial intelligence