[Image above] A “cook and look” approach to materials design can take lots of money and time—deep learning models can speed up the process immensely. Credit: Ecopetit.cat (copyright-free)
Each decade, we at ACerS look forward to seeing the new advances and discoveries the next 10 years will bring. And at the start of this time, we have fun trying to predict what areas some of the innovations will focus on.
For the 2020s, I think it is no surprise that a lot will happen in the realm of big data. Our computational storage and processing speeds have reached the point that we can tackle giant datasets on a regular basis to, for example, predict weather (though 5G may complicate these forecasts).
To process such large amounts of data, the field of artificial intelligence (AI) has grown enormously.
AI is a branch of computer science dealing with simulation of intelligent behavior in computers. In particular, there are two main subsets of AI that you’ve likely heard about in the news: machine learning and deep learning.
Machine learning is the study of computer algorithms that improve (“learn”) automatically through experience, without using a complex set of different rules. Machine learning programs are mainly based on training a model from datasets.
Deep learning, on the other hand, takes AI a step further. Its structure is inspired by our brain’s own network of neurons and is capable of learning unsupervised from data that is unstructured or unlabeled.
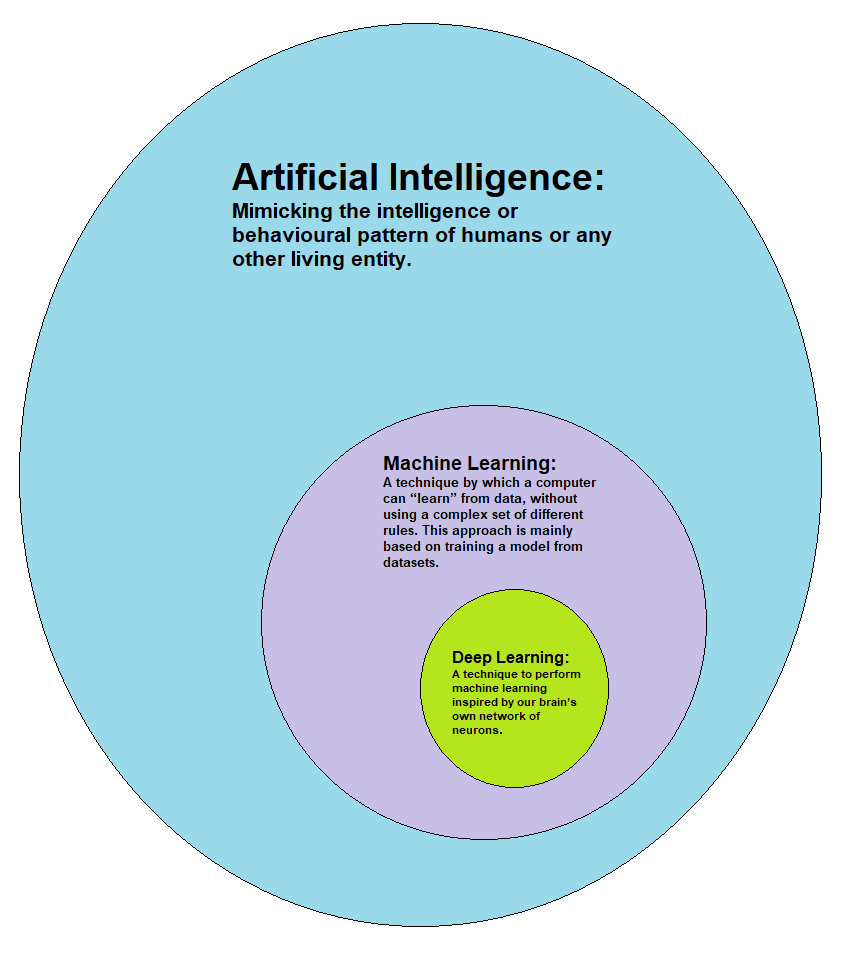
Illustration showing how artificial intelligence, machine learning, and deep learning fit together. Credit: Avimanyu786, Wikimedia (CC BY-SA 4.0)
In the past, we’ve shown several ways that big data and AI-based algorithms have furthered materials science. For example, a data-mined structure-predictor (DMSP) algorithm helped researchers predict hundreds of new stable ternary nitride compounds, and an artificial neural network helped researchers predict the Tg of oxide glasses containing anywhere from three to 21 elements.
This year, a new study harnessing the most advanced form of AI—deep learning—is poised to substantially aid in design of oxide glasses.
Oxide glass: Widespread usage, impeded discovery
Oxide glasses are an integral part of our modern world—from electronic screens and kitchenware to energy materials and bioactive implants, oxide glasses serve society in many different ways.
Because of their compositional flexibility, oxides of nearly any element can form a glass when added to glass-forming oxides. This flexibility is wonderful in that it offers seemingly limitless composition possibilities. However, the main “cook and look” approach to discovering compositions is far less desirable, as it is extremely cost, time, and resource intensive.
Recent studies on data-driven modeling of oxide glasses have shown promise in predicting composition–property relationships in glasses. But most of these studies focus on only one or two properties, “making them restrictive and, in practice, unusable for glass design, where a combination of properties is desired,” N. M. Anoop Krishnan, assistant professor in civil engineering and materials science and engineering at the Indian Institute of Technology Delhi (IIT-D) and principal investigator of the new study, says in an email.
The researchers, from IIT-D, thus looked to develop a far more encompassing modeling algorithm, one that could predict numerous glass properties and also provide two-way maps of oxide glass composition–property space.
Deep learning aids oxide glass design
To create their deep learning-based rational design paradigm, the researchers chose 37 oxide components and more than 100,000 glass compositions, encompassing glasses used for a wide range of commercial applications. They used these datasets to develop composition–property deep learning models for eight key properties:
- Density
- Young’s modulus
- Shear modulus
- Vickers hardness
- Glass transition temperature (Tg)
- Thermal expansion coefficient (TEC)
- Liquidus temperature
- Refractive index
“These properties are highly relevant for glass design—for instance, maximizing the Young’s modulus to density ratio along with maximum hardness is a criterion that is relevant for developing light-weight, scratch-resistant glasses,” R. Ravinder, research scholar at IIT-D and first author of the paper, explains in an email.
In the paper, the researchers gave details on how they developed the models, from choosing input components by performing least angle regression to using deep neural networks with hyperparametric optimization to reduce model complexity.
Based on these models, the researchers developed multi-property glass selection charts, similar to Ashby plots, that will serve as look-up charts for designing glasses with optimized compositions.
“The glass selection charts provide insights into the relationship between different properties for each glass family, thus enabling economical and rational design of oxide glasses,” Krishnan says.
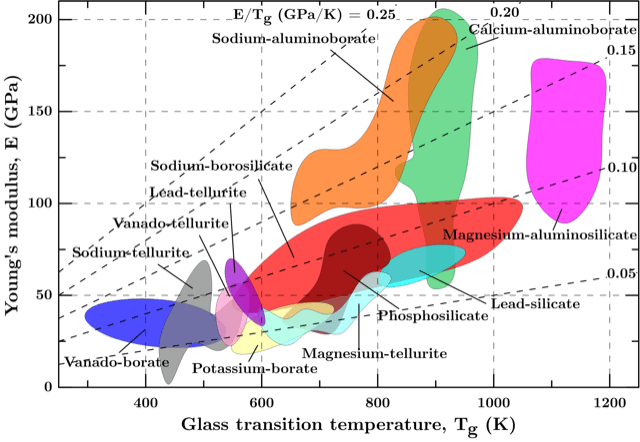
Example of a glass selection chart showing Young’s modulus with glass transition temperature for numerous commonly used glasses. Image courtesy N. M. Anoop Krishnan. Credit: R. Ravinder et al., Materials Horizons, 2020; Reproduced by permission of The Royal Society of Chemistry
After completing the models and charts, the researchers did something even more exciting—they developed a graphical user interface-based software package named Python for Glass Genomics (PyGGi), which they made publicly available online!
“PyGGi will be constantly updated and upgraded to meet the industrial and academic challenges in the field of glass science,” Hariprasad Kodamana, chemical engineering professor at IIT-D and one of the co-investigators of PyGGi, explains in a The Pioneer article. “We are also open to developing raw modules based on user requirements.”
For more information on PyGGi, contact infopyggi@iitd.ac.in.
The paper, published in Materials Horizons, is “Deep learning aided rational design of oxide glasses” (DOI: 10.1039/D0MH00162G).
The PyGGi software is available at https://pyggi.iitd.ac.in.
Author
Lisa McDonald
CTT Categories
- Basic Science
- Glass
- Modeling & Simulation