[Image above] Credit: Pixabay
Glass has played an extensive role in the lives of humans for over two millennia, from established applications such as kitchenware and windows to emerging applications such as bioactive implants and energy materials. However, despite the well-established history of this material, very few glasses among the total possible glass compositions have been discovered, and even fewer have been well-studied.
The traditional trial-and-error methodology for discovering glasses is the main reason for the limited knowledge on glass compositions. It is highly time-consuming, inefficient, and can be risky for industrial applications (for example, it may not produce the desired result).
Fortunately, recent advances in computer hardware and algorithms have led to the use of artificial intelligence (AI) and machine learning (ML) to aid in glass discovery and design. Artificial intelligence is a branch of computer science dealing with simulation of intelligent behavior in computers. Machine learning, a subset of AI, focuses on developing algorithms that can detect and understand patterns in data and extrapolate to previously unexplored domains and circumstances.
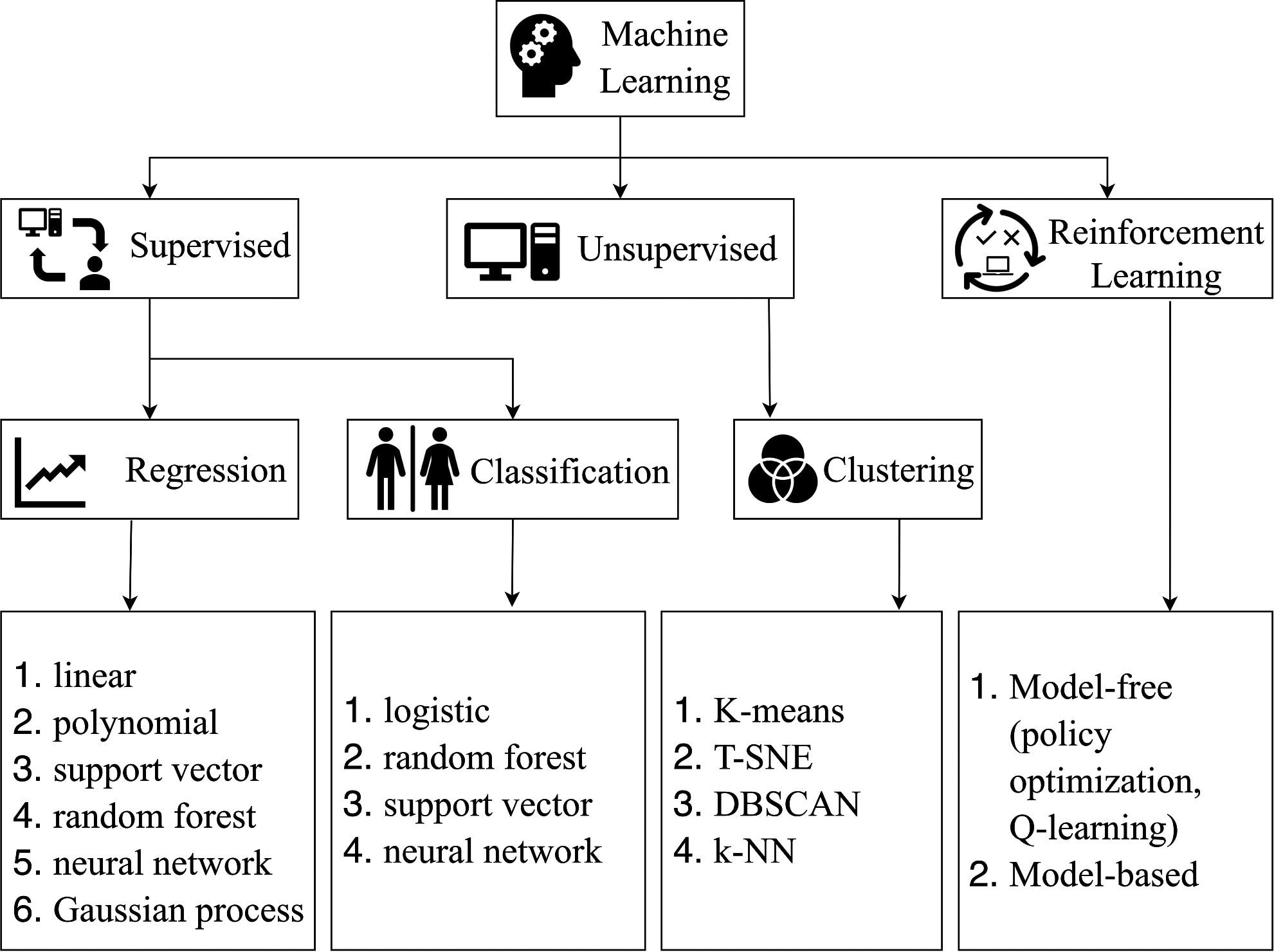
Basic paradigm for types of machine learning approaches. Credit: Ravinder et al., International Journal of Applied Glass Science
AI and ML approaches to materials design work well for glasses for several reasons, including the fact that the properties of glasses are mainly driven by composition due to their disordered structure. Thus, by applying AI/ML methods to large experimental databases of glass properties, researchers can in theory predict desirable new glass compositions quite easily.
Numerous research groups have developed models based on AI and ML to predict various properties of glass, including Young’s modulus, solubility, and glass-transition temperature, among others. However, while these studies represent great strides in using AI and ML for glass science, challenges still exist to harnessing the full potential of these methods for discovering and designing new glasses—challenges that are outlined in a new paper published in International Journal of Applied Glass Science.
The researchers who wrote the recent paper are led by N. M. Anoop Krishnan, assistant professor in civil engineering and materials science and engineering at the Indian Institute of Technology Delhi (IIT-D). In an email, Krishnan says they aimed to take a step back and look at the field of glass science and technology in a holistic manner to identify the major challenges and solutions that are enabling or are enabled by AI and ML.
Krishnan says while the challenges they identified are not an exhaustive list, they “hope that the broad areas identified will instill enthusiasm to initiate a coordinated effort from the glass community to solve some of these challenges and also identify new challenges, ultimately accelerating the field of glass science.”
The challenges identified in the paper broadly belong to two categories:
- Challenges that can be addressed using AI and ML techniques.
- Challenges that enable application of AI and ML techniques for accelerated glass design, discovery, and manufacturing.
Below is a brief overview of the 21 challenges identified in the paper.
Challenges 1–4: Developing high-quality datasets
The predictions made by AI and ML models are only as good as the data on which the predictions are based. Thus, the authors begin their list by suggesting several ways to develop high-quality datasets, including
- Automating extraction of datasets from the literature,
- Improving detection of outliers in the data, and
- Developing consistent synthetic datasets, i.e., data generated from atomistic and first-principle simulations.
Challenges 5–12: Preparing and understanding algorithms
Having developed a high-quality dataset, the next group of challenges pertain to the algorithms used to analyze that data. The authors discuss numerous aspects that researchers should be aware of when designing algorithms, including
- Selecting the right input feature,
- Selecting relevant training algorithms, and
- Selecting appropriate hyperparameters.
The authors also emphasize the importance of infusing “common-sense” into the model by accounting for basic physical laws and to quantify uncertainty of the predictions. In addition, current ML methods are “notoriously known as black-box methods” due to the difficulty of interpreting the nature of the input–output relationships, so shedding more light on this process would be extremely beneficial.
Challenges 13–17: Disseminating knowledge in the field of glass science
To date, the AI/ML models for investigating composition–property relationships typically use simple composition-based descriptors because additional information that plays a crucial role in governing the properties—such as glass preparation protocols, testing methods, and environmental conditions—often are ignored or reported in a form that cannot be easily extracted from the text. The authors offer a few ways to address these challenges to knowledge dissemination, including
- Considering how to apply knowledge learned in one area to another area;
- Identifying appropriate keywords to facilitate discovery of the study;
- Briefly summarizing a study in a holistic manner, including images, text, and chemical species present;
- Reporting on and extracting synthesis parameters of the glasses in the dataset; and
- Creating a curated image library for the glasses from literature.
Challenges 18–21: Automating the process
The authors end their list by identifying several ways to streamline glass discovery and design by automating various steps of the process, including
- Automating the glass synthesis process,
- Automating scheduling of tasks,
- Automating detection of flaws during synthesis, and
- Automating warning and safety systems for glass industries.
Krishnan says his group is already working on addressing some of these challenges. For example, they developed a software package called Python for Glass Genomics that addresses at least three of the challenges:
- Glass database from literature (PyGGi Bank).
- Machine learned composition–property models (PyGGi Seer).
- Optimized design of glasses (PyGGi Zen).
“We have also started efforts toward the application of natural language process (NLP) to address some of the challenges, such as: (i) automated extraction of glass data, (ii) information extraction from scientific literature and images, and (iii) development of image repository. A preprint from our group which addresses some of these challenges can be found at https://arxiv.org/abs/2101.01508,” he says.
The paper, published in International Journal of Applied Glass Science, is “Artificial intelligence and machine learning in glass science and technology: 21 challenges for the 21st century” (DOI: 10.1111/ijag.15881).
Author
Lisa McDonald
CTT Categories
- Education
- Glass
- Modeling & Simulation