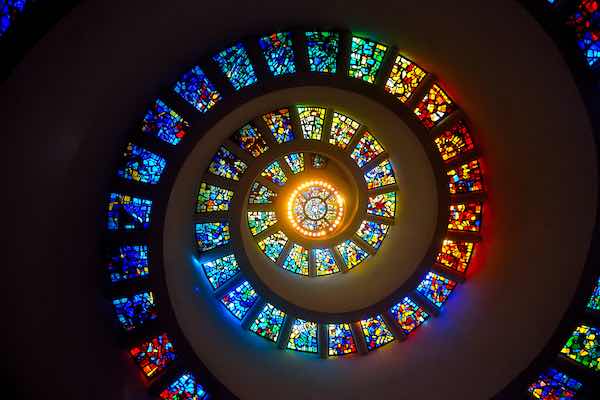
[Image above] Credit: Steve Grant; Flickr CC BY-NC-ND 2.0
In 2003, the Human Genome Project succeeded on its ambitious goal of sequencing the roughly 3 billion DNA base pairs that comprise the human genome.
The project not only unveiled humans’ genetic blueprint—opening up unprecedented possibilities in biomedical and genetics research—but it also catalyzed a new, comprehensive way of thinking about other branches of science, too.
Advances in data analysis techniques have only improved since that time, allowing the genome approach to extend its reach even deeper.
Now we have the Materials Genome Initiative, for example—a White House-backed effort “to discover, manufacture, and deploy advanced materials twice as fast, at a fraction of the cost.”
And according to a new paper published in Chemistry of Materials, scientists are developing a glass-specific genome that will allow exploration and tailoring of specific properties of glass.
Because despite a long history and a deep societal connection with glass, there’s still a lot we don’t understand about this unique and incredible material. Particularly, understanding how glass structure affects its macroscopic properties remains a significant challenge.
That’s because glass is no simple material—the evolution of glass’s structure is a complex process, extending across orders and length scales; glass compositions can widely vary and can incorporate most elements in the periodic table; and, when it comes to commercial production, manufacturers have to consider both the properties of the final glass and properties of the glass-forming liquid.
But despite those challenges, designing new functional glasses is a really important part of realizing new technologies that push the limits of conventional materials. That’s why ACerS was awarded a NIST AMTech grant last year to develop a roadmap and form a consortium to identify key needs in developing new functional glasses.
And in another effort to unveil the mysteries of glasses, scientists at Corning Inc. (Corning, N.Y.) and Aalborg University (Aalborg, Denmark) have turned to computer modeling to help decode the glass genome.
“We have a good understanding of the basic physics of many glass properties,” John Mauro, an author of the new paper and senior research manager at Corning, writes via email. “However, for other properties a solid physical understanding is still lacking.”
To try to fill that knowledge gap, the scientists examined how various models could help accurately predict how specific glass compositions will behave, and how those models can, in turn, help design glasses with optimized properties.
The authors—Mauro, Adama Tandia, K. Deenamma Vargheese, and Yihong Z. Mauro from Corning, and Morten M. Smedskjaer from Aalborg University—focused on glass produced via a fusion draw process, exploring how models of glass viscosity, refractory compatibility, liquidus temperature, diffusivity, compressive states, and elastic moduli can together aid data-driven design of superior glass compositions.
“We are very pragmatic in our modeling approach, taking advantage of physical insights wherever possible and supplementing with empirical models and machine learning to offer accurate prediction of the full suite of properties that we care about,” Mauro explains.
The results represent a step away from the trial-and-error approach to a more data-informed design strategy—which offers many benefits.
“Making use of modeling, we can design new glass compositions better, faster, and cheaper,” Mauro says. “Plus, it’s a lot of fun!”
That’s because the models will allow scientists to investigate completely unexplored composition spaces and predict their potentially unique properties—which can ultimately lead to the manufacture of new functional glasses.
But, of course, “the success of data-driven models relies on the quality of the data used as input,” Mauro says.
Moving forward, “a major thrust will be constructing a glass database with high levels of quality control needed for accurate modeling. Another big step will be to use machine learning to uncover trends and relationships among glass compositions and properties that have not yet been discovered,” Mauro adds. “Data-driven modeling can thereby be used in a ‘reverse’ sense as a tool to help guide new physical insights.”
The paper, published in Chemistry of Materials, is “Accelerating the design of functional glasses through modeling” (DOI: 10.1021/acs.chemmater.6b01054).