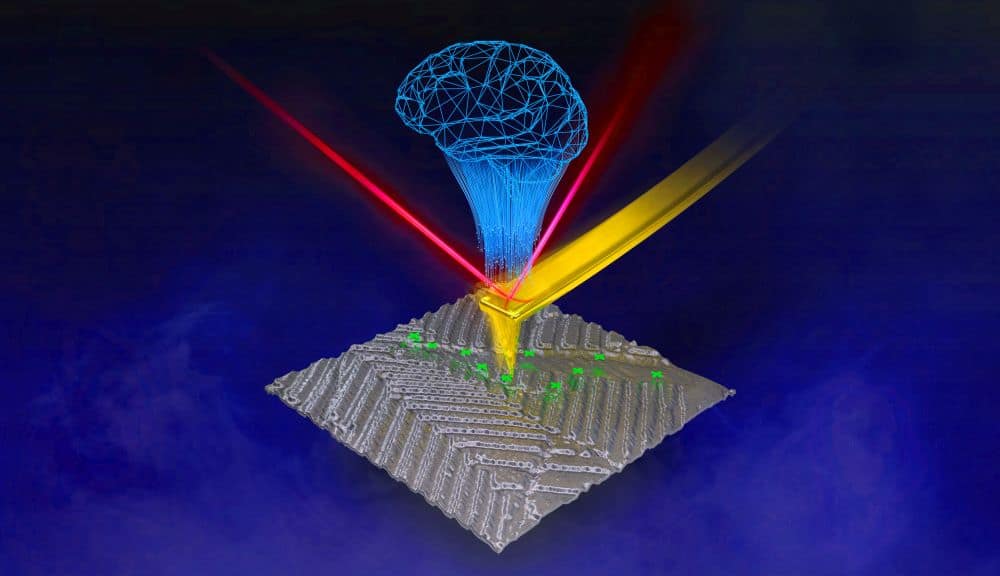
[Image above] Two recent papers offer interesting approaches to accessing, interpreting, and acting on the avalanche of data generated by sophisticated microscopy instruments. Credit: Adam Malin, Oak Ridge National Laboratory
Sophisticated instruments such as transmission electron microscopes are now standard tools in the research quiver. However, mastering the art of transmission electron microscopy takes many years of study and practice. And with these instruments costing $5–7 million each, research institutions are understandably careful about who uses them.
When used skillfully, TEMs are well worth the investment—they generate enormous amounts of information. A single eight-hour session on an aberration-corrected TEM can generate a terabyte of data, a number which can be hard to imagine.
To visualize the magnitude of this data, Lehigh University professor and ACerS Fellow Martin Harmer compares it to a stack of paper, each with a 1-MB image on it. For reference, a typical image taken with a cell phone is about 1 MB. By Harmer’s calculations, a 1-TB stack of 1-MB images printed on your office printer would be 2,640 feet tall, which is 864 feet taller than the One World Trade Center in New York City. If you aren’t calibrated by skyscrapers, think of a terabyte of data as 500 hours of movies, or 200,000 five-minute songs.
Today’s hard drives offer terabyte-scale storage capability, so storing that much data is actually quite easy.
Unfortunately, the capacity of the human brain—marvelous though it is—has not expanded with the explosion of data, and accessing, interpreting, and acting on that much data is not so easy for the human brain.
So, how does a researcher begin to work with so much data? Is much discovery lost in the data avalanche, and are there strategies for managing it?
Thankfully, the human brain is very clever at dealing with its limitations. Two recent papers offer interesting approaches to this challenge.
Optimizing the human–instrument interface
At Lehigh University, Harmer leads the Presidential Nano-Human Interfaces (NHI) Initiative to rethink the interaction between human researchers and their scientific instruments.
Microscopists typically sit at a TEM’s control panel, turning knobs to find and examine tiny areas of interest. But what if the human–TEM instrument interface could be automated and optimized, similar to the way a fighter jet cockpit panel is optimized to allow the pilot to focus on the mission with minimal distraction from the business of flying the jet? What is gained—or lost—by having the researcher interact with the instrument through a remote computer interface that would take over some of the “flying the machine” tasks?
To understand the challenges and consequences of adopting a remote, computer-aided instrument interaction, researchers in the NHI Initiative turned to cognitive science to learn about skills essential to microscopy, such as spatial reasoning, procedural memory, judgement and decision-making, and new skills acquisition.
In a November 2021 MRS Bulletin opinion paper, the Lehigh group of microscopists and cognitive scientists reported on an experiment to understand how microscopists at different skill levels interact with TEMs directly and remotely and how that translates to image quality.
The team used head-mounted, eye-tracking equipment to observe three students with instrument proficiency at the novice, intermediate, and expert levels. Two modalities were observed: direct interaction with the instrument’s control panel and interaction via a modified semi-remote setup, where the signal is recorded and shown on a separate computer screen.
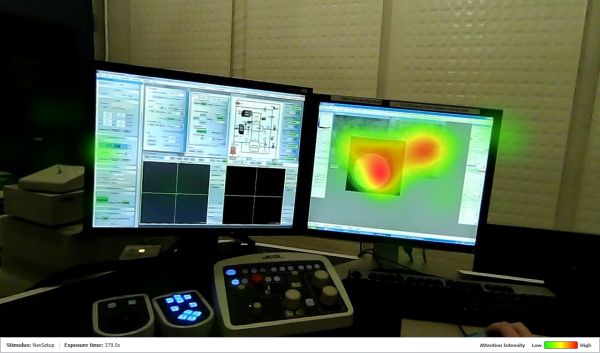
The team measured time spent on three key microscopy tasks: navigating to the sample area of interest, focusing and optimizing the image, and acquiring/saving the image.
All three skill levels acquired and saved images with about the same speed and proficiency. However, while it took all three skill levels longer to achieve navigation and focusing tasks in the remote configuration, there were some surprises.
The beginner took longer for both tasks than the intermediate user, as might be expected. Surprisingly, the expert was much slower than expected when operating the instrument in the remote configuration compared to the direct interaction. The expert needed 20 minutes more to achieve tasks remotely compared to direct interaction with the instrument, whereas the beginner and intermediate microscopists took only five minutes more to achieve the tasks in the remote configuration.
But more important than time is the quality of the image. The beginner produced the least useful images in both direct and remote configurations. The expert produced the highest quality images when operating the instrument directly. However, the intermediate user produced the highest quality images of the group when working in the remote configuration, albeit not as high quality as the expert’s image when controlling the instrument directly.
The bottom line is that there is a “performance cost” associated with a transition away from direct control of the instrument to a remote configuration, and it will impact expert microscopists more than microscopists who are still gaining their skills. In the paper, the authors note that “individuals with vast experience in a single user interaction may assume that such usability changes will not affect their performance, but counterintuitively, cognitive science research on expertise suggests that individuals with more experience may suffer greater performance disruptions than a novice user might when such transitions are required.”
The field of microscopy is a good proxy for identifying the human challenges of working with any large data-generating process. The microscopists were all students, so minds are still nimble enough to “learn new tricks.” This experiment shows us there is much opportunity for the cognitive sciences to inform optimization of user interfaces, not just on fighter jets but also on TEMs, manufacturing control panels, power plant control systems, mining operations, and on and on.
The paper, published in MRS Bulletin, is “The Lehigh Presidential Nano-Human Interface Initiative: Convergence of materials and cognitive sciences,” (DOI: 10.1557/s43577-021-00232-y).
Let the material do the driving?
A research group at Oak Ridge National Laboratory is also thinking about microscopy and how to use it to discover new materials.
Functional materials, they realized, could help guide decisions leading to discovery because these materials respond to external stimuli, such as applied fields. If the researchers could write algorithms with proper workflows, they could teach the instrument how to automatically probe the material. The instrument would learn from the material’s response and automatically take the next step in a microscopy session.
Would it be possible to develop a workflow for machine learning to accelerate characterization that would lead to new materials discovery?
A recent paper published in Nature Machine Intelligence reports on the development and implementation of a machine learning framework based on the known characteristics of the ferroelectric hysteresis loop.
Autonomous microscopy sounds simple but requires the solution of three problems: controlling the microscope with external electronics, developing the machine learning algorithms to run automated experiments, and identifying the problem(s) for the automated experiment to solve. In the paper, the authors note that the third issue—“what is the experiment”—has not received as much attention as the other two challenges, an issue this paper aimed to rectify.
In a press release, ORNL researcher Maxim Ziatdinov says, “We can use smart automation to access unexplored materials as well as create a shareable, reproducible path to discoveries that have not previously been possible.”
The team used scanning probe microscopy to test their idea on a ferroelectric PbTiO3 thin film deposited via metal organic chemical vapor deposition on a KTaO3 substrate with a SrRuO3 buffer layer. They turned to deep kernel learning to build an experimental workflow to probe the material’s structure–property relationships, specifically, the relationship between local domain structure and the hysteresis loop.
“We wanted to move away from training computers exclusively on data from previous experiments and instead teach computers how to think like researchers and learn on the fly,” says Ziatdinov in the press release. “Our approach is inspired by human intuition and recognizes that many material discoveries have been made through the trial and error of researchers who rely on their expertise and experience to guess where to look.”
The workflow developed by the team produced results faster by orders of magnitude than conventional workflows, and it points the way to new advances in automated microscopy.
“The takeaway is that the workflow was applied to material systems familiar to the research community and made a fundamental finding, something not previously known, very quickly—in this case, within a few hours,” Ziatdinov says.
In the preprint version of the paper, the team notes that the machine learning approach is extensible to other structure–property modes, and that it may be especially beneficial in situations where the data acquisition times are large, measurements are destructive (e.g., nanoindentation), or irreversible experiments (such as electrochemical experiments).
The paper, published in Nature Machine Intelligence, is “Experimental discovery of structure–property relationships in ferroelectric materials via active learning,” (DOI: 10.1038/s42256-022-00460-0).