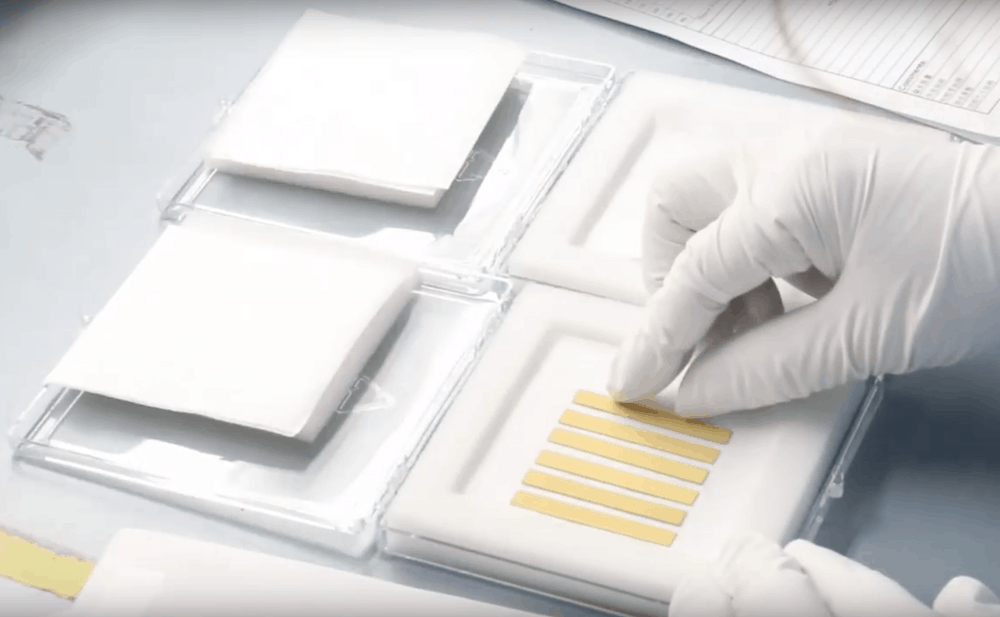
[Image above] Video tour of CTS Corporation’s state-of-the-art piezoelectric facility in Tianjin, China. Many manufacturers use modeling to identify new piezoelectric materials. Credit: CTS Corporation, YouTube
With an estimated $33.9 billion market value by 2024, piezoelectric devices are a quickly growing market segment that play an important role in sensing, actuating, and signal processing applications.
Piezoelectric devices are ones that make use of the piezoelectric effect—the ability to generate an electrical charge in response to applied mechanical stress and vice versa. Researchers frequently use models to evaluate the performance of new piezoelectric materials to cut down on the expense of testing everything through experiment.
“However, the accuracy of these measures is limited by knowledge of the material properties. Thus, it is necessary to use various techniques to determine the parameters for the piezoelectric constitutive equations,” two researchers write in a recent open-access paper.
The researchers are professors Mariana del Castillo and Nicolás Pérez from University of the Republic, Uruguay. In their study, they looked to improve modeling of piezoelectric materials through the use of machine learning.
They begin by explaining that the finite element method (FEM) is a widely used numerical technique for identifying piezoelectric material parameters, including elastic, piezoelectric, and dielectric constants. This iterative procedure takes time, however, which is why the researchers propose using neural networks to speed up the process.
Neural networks are a series of algorithms that aim to recognize underlying relationships in a set of data through a process that mimics the human brain. While FEM simulations are needed to train a neural network, the trained neural network “is very fast compared to the loop of [FEM],” they elaborate.
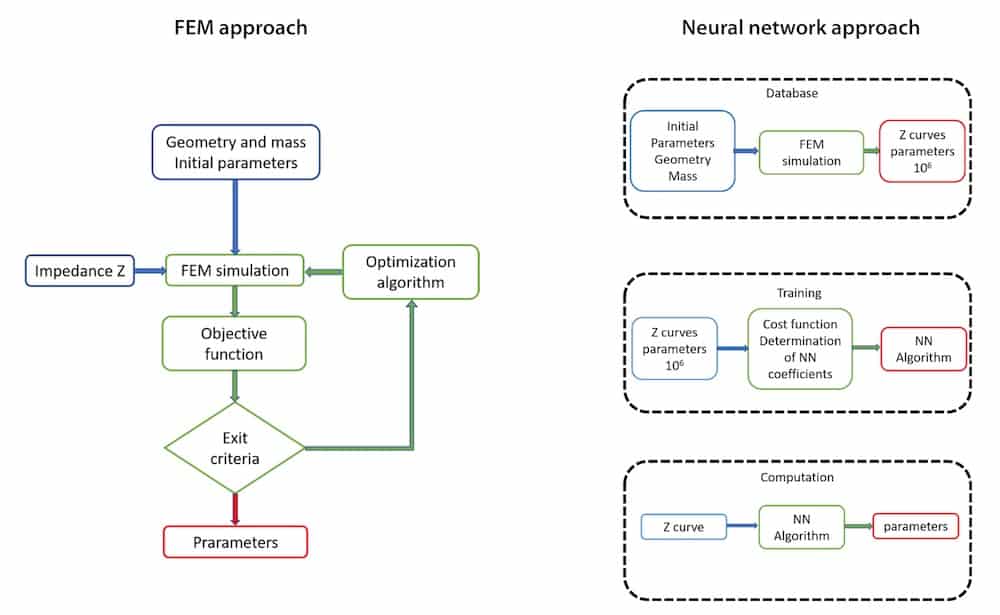
The researchers used data from the piezoelectric ceramic Pz27 from Meggitt to train the neural network. First, they generated a dataset containing 600,000 synthetic impedance curves by setting a ±10% range of variation around the original parameter values. After training on this dataset, the neural network was tested using two different sets of curves, the first restricted to the subspace of more sensitive parameters and the other with all parameters changing at random.
They found that testing conducted on the restricted subspace identified parameters suitable for use in a Nelder–Mead nonlinear minimization to obtain final results. Testing that included all parameters resulted in larger error but was still suitable for use.
“This first contribution shows the feasibility of the technique to solve the problem. Next steps include the generalization for the full parameter set and testing the application with real piezoceramics,” they conclude.
The open-access paper, published in Materials, is “Machine learning identification of piezoelectric properties” (DOI: 10.3390/ma14092405).