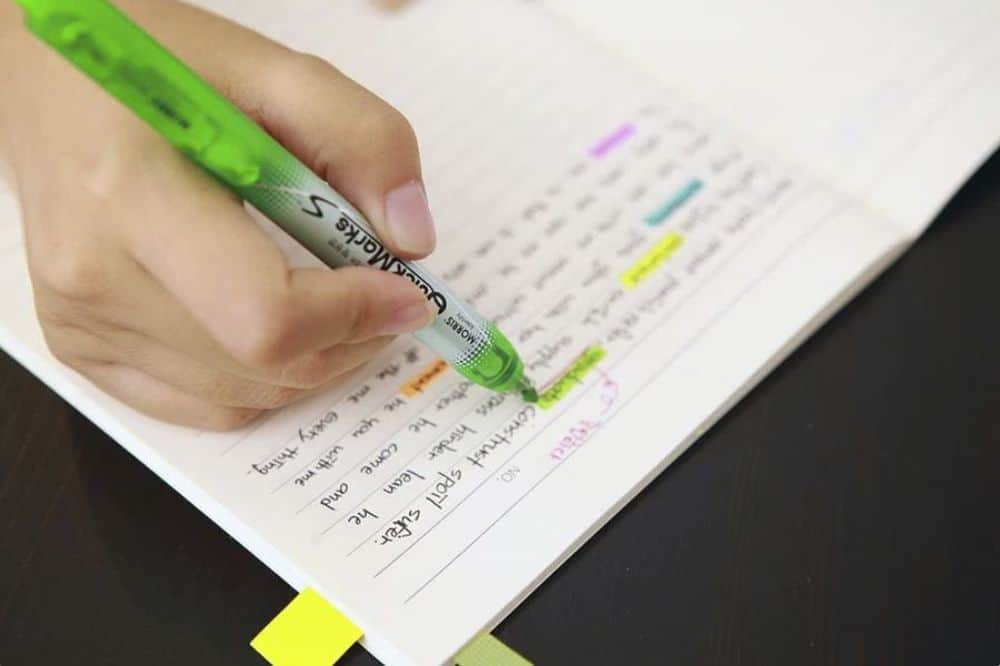
[Image above] Credit: rawpixel (CC0)
As countries around the world stumble their way toward implementing effective and equitable open-access policies, the public availability of data is only the first step in making scientific research accessible to everyone. People will also need ways to sort and categorize these vast swaths of data so insightful patterns and conclusions can be identified and extracted.
This need is particularly pressing in the materials science community. Currently, most existing databases for material properties contain calculated rather than experimental values due to the nonuniform presentation of data across scientific texts, which makes data extraction difficult. Calculated values may not accurately reflect real-world scenarios, however, so curating experimental data lays the groundwork for more accurate analysis and comparison.
The emergence of large language models, such as those that power ChatGPT, have the potential to revolutionize data extraction and curation. These systems are trained to achieve general-purpose language understanding, which allows them to automatically extract relevant data from scientific literature.
Extracting the relevant data can only happen after the text is properly labeled and organized, however. And as mentioned above, scientific texts typically do not present data in a structured, uniform manner. So, researchers are often left with the cumbersome task of annotating the data before extraction.
Several groups have explored the development of semi-automatic annotation methods to reduce the need for manual labeling, such as here, here, and here. Inspired by these past works, researchers led by University of Utah associate professor Taylor Sparks developed a new method that harnesses the power of Google’s Gemini Pro language model to further reduce the need for manual annotation.
As explained in the paper describing their new method, Sparks and his graduate students Hasan M. Sayeed and Trupti Mohanty fed text from materials science literature into the model one section at a time. They chose this approach “to ensure that similar kinds of information remain together, enhancing the clarity and coherence of the annotations,” they explain.
They selected a diverse set of 10 articles spanning various domains within materials science, such as supercapacitors, high-entropy alloys, batteries, and ceramics, to test their semi-automatic annotation method. Compared to manual annotation of the papers, which typically requires about 55 minutes per paper, the use of Gemini Pro reduced the need for manual editing and corrections to about 15 minutes per paper.
“This demonstrates the potential for our approach to minimize manual effort while ensuring high-quality annotations, making it a promising solution for accelerating data extraction from materials science literature,” they write.
In the paper’s discussion section, Sparks and his students note that while their method used only a single language model, it is worth considering the use of multiple models for benchmarking purposes. Additionally, future research may explore using a large language model that is fine-tuned for materials science literature, unlike Gemini Pro.
“By pursuing these avenues for improvement, we can continue to advance semi-automated annotation techniques,” they conclude.
Readers interested in accessing the annotated data generated in this study can access it on Figshare.
The paper, published in Integrating Materials and Manufacturing Innovation, is “Annotating materials science text: A semi-automated approach for crafting outputs with Gemini Pro” (DOI: 10.1007/s40192-024-00356-4).