[Image above] A new materials characterization method is useful for analyzing data from a variety of sources and analyzing multiple data sets simultaneously. Credit: NC State
It’s kind of amazing to think that all the stuff around us—from lithium-ions to livers to lichens to lunchboxes—is composed of a relatively small number of atomic ingredients.
Atoms come in just 118 identified elemental flavors, yet they yield enough possible combinations to give us stuff that spans the entire adjective gamut.
So understanding how those atoms are combined and structured together is really important when it comes to understanding the resulting materials’ properties and, perhaps even more so, for predicting the properties of new materials.
And for accurate predictions, we need good models.
So researchers at North Carolina State University (Raleigh, N.C.), the National Institute of Standards and Technology (Gaithersburg, Md.), and Oak Ridge National Laboratory (Oak Ridge, Tenn.) have devised a new method for characterizing materials that can more accurately predict a material’s crystallographic structure.
“This approach will allow us to analyze data from a wide variety of materials characterization techniques—all forms of spectroscopy, mass spectrometry, you name it—and more fully characterize all kinds of matter,” ACerS Fellow Jacob Jones, a professor of materials science and engineering at NC State and co-author of a paper on the work, says in a NC State news release. “Honestly, it’s very exciting.”
So what’s the secret to new method? Bayesian statistics.
Experiments to characterize materials usually make inferences from diffraction experiments. But the problem is that those inferences typically are made using oversimplified statistical models. Bayesian statistics, however, can provide a richer solution.
The oversimplified models make assumptions about a material based on a single unit cell, and then extrapolate that unit cell across the entire material to predicts its overall properties. While such analysis is sufficient, it’s really not very accurate.
That’s because models from a single unit cell uses least squares analysis to estimate parameters of the structure, such as atomic positions and lattice constants, as single values—even though we know that these parameters are heterogeneous across a material.
In other words, materials aren’t composed of perfectly uniform unit cells.
The power in the new Bayesian-based method is that it doesn’t reduce these parameters to single values—it gives them wiggle room. The method represents structural parameters, such as atomic distance, as probability distributions, which provide a richer prediction of a material’s crystallographic structure.
“For example, atoms vibrate,” Alyson Wilson, an NC State professor of statistics and co-author of the new paper, says in a NC State news release. “And the extent of the vibration is controlled by their temperature. Researchers want to know how those vibrations are influenced by temperature for any given material. And Bayesian tools can give us probabilities of these thermal displacements in a material.”
Using probabilities, instead of single values, provides not only a better prediction of a material’s structure overall, but also a better prediction of how a material will perform during applications and a better way of qualifying manufactured materials, Jones explains.
And part of the incredible power of this new technique—beyond its ability to more accurately predict atomic positions—is its wide applicability because of the ubiquity of these types of experiments to characterize nearly all materials.
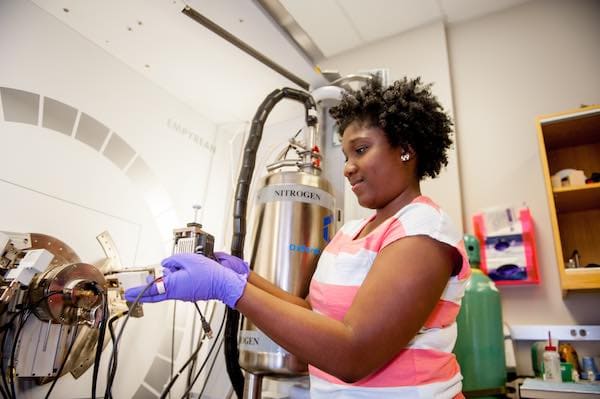
NC State graduate student Brienne Johnson performs an X-ray diffraction experiment. Credit: NC State
But Jones also says the method may be particularly useful for characterizing certain types of materials. The new method is exceptionally useful for combining different types of data, so materials that require various characterization experiments now have a better model to predict their structures.
For example, metal oxides often require neutron diffraction experiments because they provide better sensitivity to light elements in the presence of heavy elements, Jones explains. But high-resolution X-ray diffraction can describe these materials’ lattice constants more accurately, so ideally a researcher would combine both methods to more accurately describe metal oxides.
The new analysis method can then integrate these different types of data, and other types of data, too, to provide a much more thorough analysis of the metal oxides’ crystallographic structure.
Beyond helping predict and design new materials, however, the method has industry-specific applications, too.
For example, material suppliers of ceramic particles could use the method to more accurately quantify the size distributions of their products, providing a range of particle sizes rather than an average particle size, Jones says.
“However, one remaining challenge is to develop software that can incorporate this method into evaluations of diffraction and spectroscopy data” Jones says. “Specialists can write the codes, but we need to make them widely available to industry.”
The open-access paper, published in Scientific Reports, is “Use of Bayesian inference in crystallographic structure refinement via full diffraction profile analysis” (DOI: 10.1038/srep31625).
Author
April Gocha
CTT Categories
- Basic Science
- Material Innovations
- Modeling & Simulation