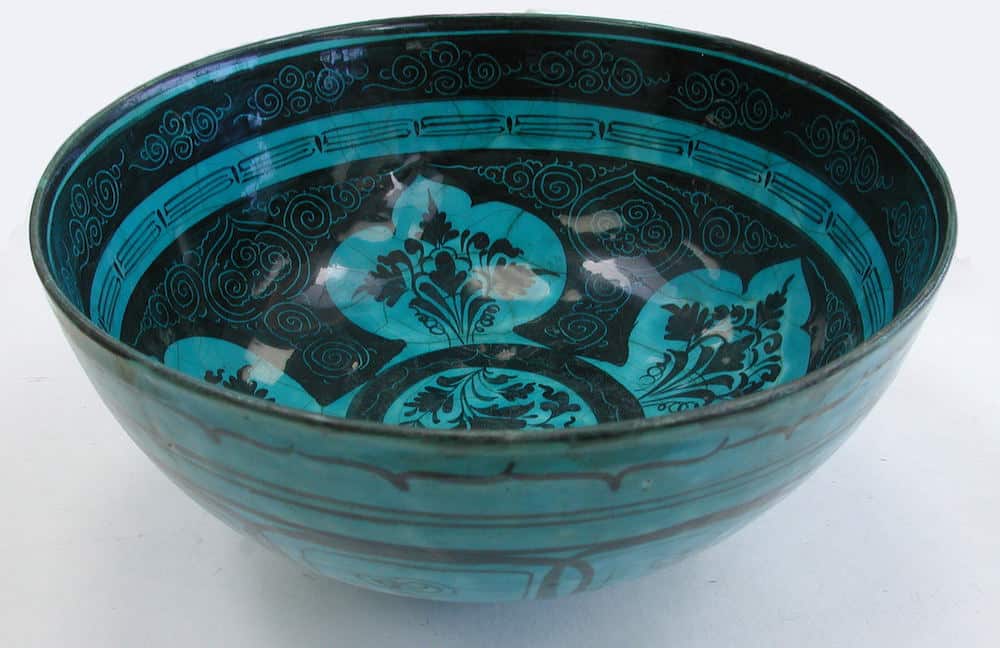
[Image above] This striking turquoise and black bowl is an example of Kubachi ware, a type of Persian artwork. Two researchers in Iran developed a deep learning method to help restore this type of artwork. Credit: The Met Fifth Avenue
When we cover development of advanced techniques on CTT, we often consider how these technologies will move us toward the future. But these technologies can be useful for bringing the past back to life as well.
For example, we’ve taken a couple looks at how ceramic additive manufacturing is demonstrating potential in artifact restoration, allowing us to appreciate how historical pieces may have looked in their former glory. But repairing the bulk of an artifact is not the only step in restoration. For the overall aesthetic aspects of a piece to be preserved, researchers must replicate the visual complexity and diversity of the historical artwork’s motifs as well.
Inpainting is the process of reconstructing damaged, deteriorated, or missing parts of an artwork using the surrounding areas as indicators of the lost motif. While the aim of inpainting is to preserve the overall aesthetic aspects of the artwork without being noticed, it can be difficult for a single artist to imagine all the patterns that will match a historical artwork’s visual complexity and diversity.
Since the mid-1990s, museum artists have used digital inpainting techniques to aid in restoration. Early techniques were non-learning based, meaning they used procedural algorithms to generate new pixels with the help of neighboring areas. Because these methods were not aware of the entire artwork, they were only suitable for restoring small holes with simple textures because otherwise blurred parts or mismatches could be generated.
In recent years, advancement of machine learning techniques has significantly improved the performance of automatic image understanding by allowing a program to consider the entire artwork when generating patterns. The drawback to using multilayered artificial neural networks for digital inpainting, however, is the extensive time required to build the model.
To overcome this burden, researchers have looked at convolutional neural networks (CNNs) with a shared-weights architecture to reduce the number of links between layers and thus speed up the modeling process.
There are several studies on using CNNs for inpainting applications. For example, one work used the idea of encoding-decoding architecture, and another paper proposed an architecture with both global and local consistency.
Almost all proposed CNN-based inpainting techniques require some post-processing steps to refine the final output and reduce visual artifacts created during the prediction. In addition, most assume that the missing parts are located in the center of the image with a rectangular shape, which is not an acceptable assumption for historical artworks for several reasons.
To tackle these drawbacks, a group of researchers in 2018 proposed a modified convolutional layer that considers only valid pixels when developing its prediction. Experimental results from this so-called partial convolution have demonstrated the effectiveness of the method.
Motivated by these results, two researchers from Azarbaijan Shahid Madani University in Tabriz, Iran, recently published a paper to expand on this approach to CNN-based digital inpainting.
For this study, they trained the CNN model to restore a style of Persian pottery called Kubachi ware. Kubachi ware is one of the most used styles in Iranian historical artworks. This type of pottery appears mostly in the form of bowls, plates, and tiles. It is composed of a very fine and soft white material known as stone paste, and it is adorned with various ornamental motifs in colors such as black, green, blue, ocher, and brown under a transparent crackled glaze.
Scholars believe that Kubachi ware pieces were created in the northwestern part of what is now Iran during the Safavid Period (1501–1736 CE). However, this pottery style takes its name from the village of Kubachi in Dagestan, modern-day Russia, where large quantities of Kubachi pottery were discovered.
The researchers collected 660 different images of Kubachi ware and Iznik pottery pieces, which are very similar, and then extracted subimages from these images to augment the number of samples. “We should note that, as the motifs and patterns in most of the images are asymmetric, we were sure that the extracted images were almost unique,” they write.
They also photographed images of existing damaged pottery pieces around the mosques and old cultural houses in Isfahan, a city in central Iran, to evaluate the framework on real-world cases. However, due to some legal and administrative restrictions, they could only collect 17 images this way.
After evaluating the predictions generated by the CNN-based model, the researchers determined that the proposed framework restored heavily destroyed parts of Kubachi ware very well and preserved the overall aesthetic aspects quite plausibly. “However, we believe that the performance would increase if we could prepare a sufficient number of samples to feed the model,” they add.
In the future, the researchers plan to add more samples to the datasets. They also aim to extend the framework to other types of historical artworks.
The paper, published in Journal of Computational Science, is “A deep neural network based framework for restoring the damaged Persian pottery via digital inpainting” (DOI: 10.1016/j.jocs.2021.101486).