Bilge Yildiz is the Breene M. Kerr (1951) Professor in the Nuclear Science and Engineering and the Materials Science and Engineering Departments at Massachusetts Institute of Technology (MIT), where she leads the Laboratory for Electrochemical Interfaces. She received her PhD at MIT in 2003 and her BSc from Hacettepe University in 1999. After working at Argonne National Laboratory as a research scientist, she returned to MIT as an assistant professor in 2007.
Yildiz’s research focuses on laying the scientific groundwork to enable next generation electrochemical devices for energy conversion and information processing. The scientific insights derived from her research guide the design of novel materials and interfaces for efficient and durable solid oxide fuel cells, electrolytic water splitting, brain-inspired computing, and solid state batteries. Her laboratory has made significant contributions in advancing the molecular-level understanding of oxygen reduction, water splitting, ion diffusion, and charge transfer on mixed ionic-electronic conducting oxides. Her research has uncovered the effects of surface chemistry, elastic strain, dislocations, and strong electric fields on the reactivity, efficiency, and degradation in these applications. Her approach combines computational and experimental analyses of electronic structure, defect mobility and composition, using in situ scanning tunneling and X-ray spectroscopy together with first-principles calculations and novel atomistic simulations.
Yildiz’s teaching and research efforts have been recognized by the Argonne Pace Setter (2006), ANS Outstanding Teaching (2008), NSF CAREER (2011), IU-MRS Somiya (2012), the ECS Charles Tobias Young Investigator (2012), the ACerS Ross Coffin Purdy (2018), and the LG Chem Global Innovation Contest (2020) awards.
Title: Energy-efficient hardware and intelligent materials for brain-inspired Computing: Artificial synapses based on proton and oxygen motion
Abstract: Deep learning is a powerful algorithm for machine learning applications such as computer vision and natural language processing. However, the training of these neural networks is limited by the traditional von Neumann architecture of our current CPUs and GPUs. Shuttling data back and forth between the separate memory and computation units in such architecture results in significant energy consumption; many orders of magnitude greater than the energy consumption in human brain. In our research, we aim to reduce the energy consumption in analog, brain-inspired computing, by focusing on designing materials and devices that can instead perform data storage and computation in a single architecture using ions. First, I will share our work on a protonic electrochemical synapse concept that changes conductivity deterministically by current-controlled shuffling of dopant protons across the active device layer; resulting in energy consumption on par with biological synapses in the brain. Second, I will share our work in controlling the consistency of conducting oxygen vacancy filament formation in resistive memory devices via dopant and microstructure engineering. These strategies provide pathways towards brain-inspired hardware that has high yield and consistency and uses significantly lesser energy as compared to current computing architectures.
Subscribe to Ceramic Tech Today

Don’t miss the latest ceramic and glass materials news. Receive the CTT newsletter to your email three times a week by subscribing at this link.
Subscribe to Ceramic & Glass Manufacturing Weekly
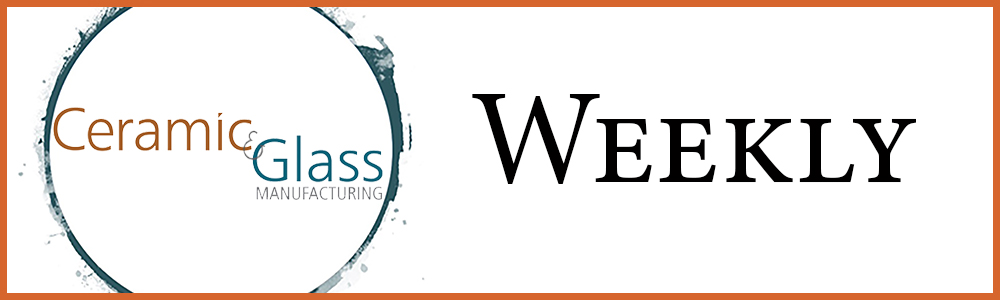
Don’t miss the latest ceramic and glass business news. Receive the C&GM Weekly newsletter to your email every Monday by subscribing at this link.